2.12 Project: Clustering Newspaper Articles
Contents
2.12 Project: Clustering Newspaper Articles#
In this lesson, we’ll see how to leverage sentence embeddings to perform clustering of newspaper articles.
The Clustering Pipeline#
Here’s what we are going to do:
Create embeddings for the newspaper articles using
sentence-transformers
.Reduce the dimensionality of the embeddings using
umap
.Visualize the embeddings with
plotly
.Clusterize the embeddings using
hdbscan
.Assign a meaningful name to each cluster leveraging keyword extraction techniques from
keybert
.
The Dataset#
The AG (Antonio Gulli) dataset is a collection of more than 1 million news articles. Here’s its description in its Hugging Face dataset card:
News articles have been gathered from more than 2000 news sources by ComeToMyHead in more than 1 year of activity. The dataset is provided by the academic comunity for research purposes in data mining (clustering, classification, etc), information retrieval (ranking, search, etc), xml, data compression, data streaming, and any other non-commercial activity.
We’ll cluster a subset of 3k articles from it.
Dimensionality Reduction with UMAP#
UMAP is a flexible non-linear dimensionality reduction algorithm based on manifold learning, very useful for visualizing high-dimensional datasets. Read this article to get an understanding of how it works and see a comparison with TSNE.
Clustering with HDBSCAN#
HDBSCAN (Hierarchical Density-Based Spatial Clustering of Applications with Noise) is a variation of the DBSCAN clustering algorithm, allowing finding clusters of varying densities (unlike DBSCAN), and being more robust to parameter selection. You can learn more about it in its documentation.
I’d suggest reading also this article about clustering methods in sklearn
to learn about commonly used clustering algorithms. The following image is taken from that article.
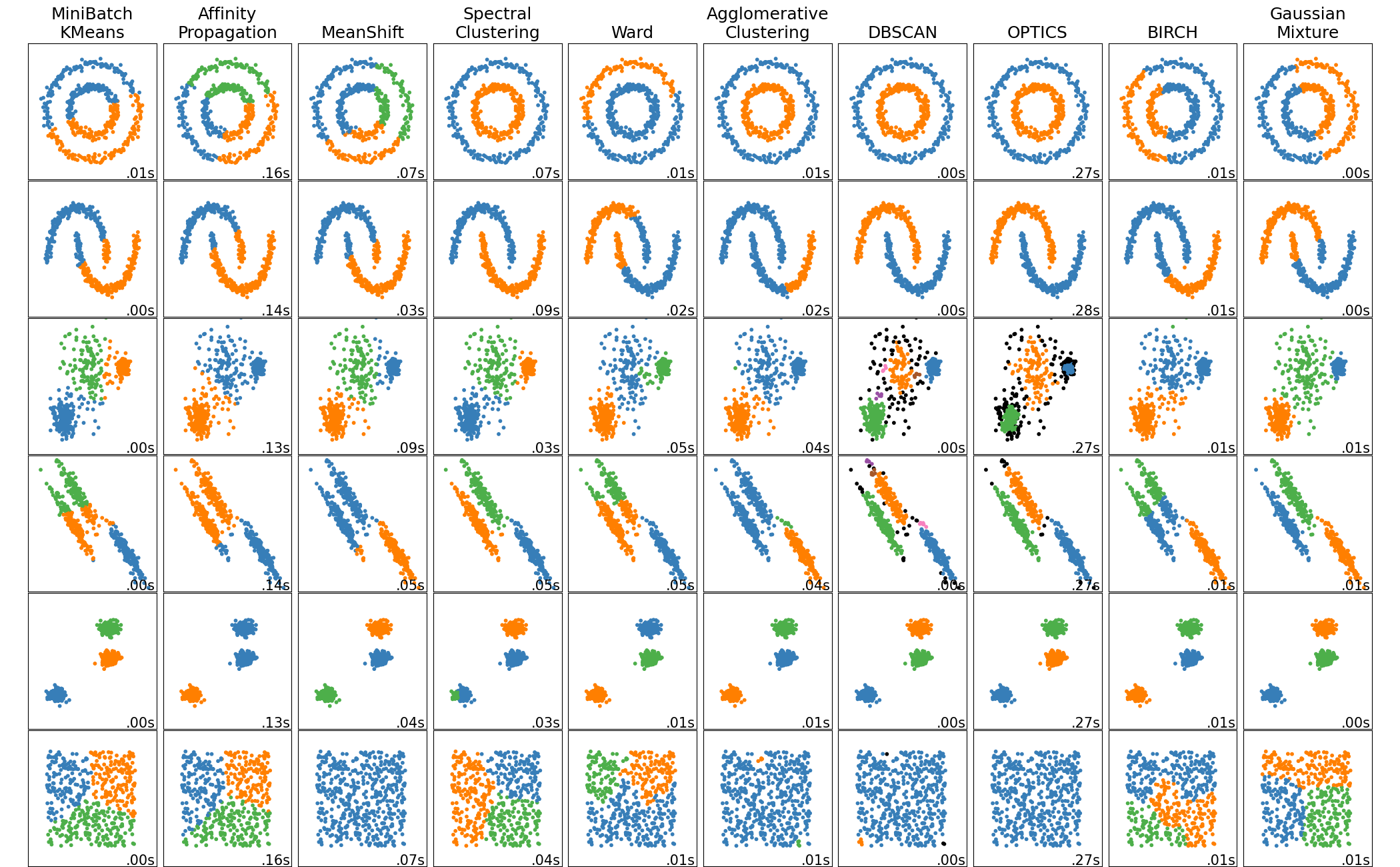
Extracting Keywords with KeyBERT#
KeyBERT is a easy-to-use library for extracting keywords from text. Here’s how it’s described in its repository:
KeyBERT is a minimal and easy-to-use keyword extraction technique that leverages BERT embeddings to create keywords and keyphrases that are most similar to a document.
In short words, the library uses BERT-embeddings and simple cosine similarity to find the sub-phrases in a document that are the most similar to the document itself. You can learn more about how it works in this Medium post.
Coding with Python#
We are now ready to implement the clustering pipeline over the AG dataset.
Install and Import Libraries#
Let’s install the necessary libraries.
pip install datasets sentence-transformers umap-learn hdbscan keybert
We’re going to use the umap-learn
library for its implementation of the UMAP dimensionality reduction algorithm, hdbscan
for its implementation of the clustering algorithm HDBSCAN, and keybert
for its keyword extraction techniques.
# manage data
from datasets import load_dataset
import pandas as pd
# embeddings
from sentence_transformers import SentenceTransformer
# dimensionality reduction
import umap
# clustering
import hdbscan
# extract keywords from texts
# used to assign meaningful names to clusters
from keybert import KeyBERT
# visualization
import plotly.express as px
Download and Prepare Dataset#
As we often do, we load the dataset using the load_dataset
function.
# download data
dataset = load_dataset("ag_news", split="train")
print(dataset)
Dataset({
features: ['text', 'label'],
num_rows: 120000
})
The dataset contains 120k articles. Let’s keep only 3k of them to make computations faster in this project.
# keep only first 3k articles to make computations faster
dataset_subset = dataset.train_test_split(train_size=3000)["train"]
print(dataset_subset)
Dataset({
features: ['text', 'label'],
num_rows: 120000
})
Then, we convert the dataset to a pandas
dataframe and drop its label
column (which is used for benchmarking text classification and it’s not our goal in this project).
# convert dataset to pandas dataframe
df = pd.DataFrame(dataset_subset).drop("label", axis=1)
df.head()
text | |
---|---|
0 | Mistakes cost Dallas dearly Mistakes will driv... |
1 | Bomb scare forces Singapore plane to UK A Sing... |
2 | Only Drills, but Houston Looks Ready to Return... |
3 | Pact to speed Navy Yard plans The Boston Redev... |
4 | Pixar's Waiting for Summer Plus, IBM's win-win... |
Create Articles Embeddings#
Let’s download an embedding model using the sentence-transformers
library.
# download the sentence embeddings model
embedder = SentenceTransformer('all-mpnet-base-v2')
We then get the embedding of all the articles in our dataset.
# embed article texts
corpus_embeddings = embedder.encode(df["text"].values)
print(corpus_embeddings.shape)
(3000, 768)
Reduce Embeddings Size#
To visualize the embeddings in two dimensions, let’s reduce their dimensions to 2 using UMAP. UMAP is rather sensible to its hyperparameters, so you can find in the code the hyperparameters that worked the best in my experiments.
# reduce the size of the embeddings using UMAP
reduced_embeddings = umap.UMAP(n_components=2, n_neighbors=100, min_dist=0.02).fit_transform(corpus_embeddings)
print(reduced_embeddings.shape)
# put the values of the two dimensions inside the dataframe
df["x"] = reduced_embeddings[:, 0]
df["y"] = reduced_embeddings[:, 1]
# substring of the full text, for visualization purposes
df["text_short"] = df["text"].str[:100]
(3000, 2)
Embeddings Visualization#
We are now ready to visualize our embeddings in 2 dimensions using plotly
.
# scatter plot
hover_data = {
"text_short": True,
"x": False,
"y": False
}
fig = px.scatter(df, x="x", y="y", template="plotly_dark",
title="Embeddings", hover_data=hover_data)
fig.update_layout(showlegend=False)
fig.show()
Clustering#
Next, let’s clusterize the reduced embeddings using HDBSCAN. To know more about its hyperparameters, read its documentation.
# clustering with HDBSCAN
clusterer = hdbscan.HDBSCAN(min_cluster_size=9)
labels = clusterer.fit_predict(reduced_embeddings)
df["label"] = [str(label) for label in labels]
print(f"Num of clusters: {labels.max()}")
Num of clusters: 43
The clusters will be named with increasing positive numbers starting from “0”. You’ll find also that several samples are labeled with “-1”, which means that they couldn’t be associated to existing clusters and so they’re outliers.
# number of outliers
num_outliers = len(df[df["label"] == "-1"])
print(f"Num of outliers: {num_outliers} ({num_outliers / len(df) * 100:.2f} % of total)")
Num of outliers: 812 (27.07 % of total)
Let’s now plot the embeddings of the articles colored by their clusters, and leaving out all the outliers.
# remove outliers
df_no_outliers = df[df["label"] != "-1"]
# scatter plot
hover_data = {
"text_short": True,
"x": False,
"y": False
}
fig = px.scatter(df_no_outliers, x="x", y="y", template="plotly_dark",
title="Embeddings", color="label", hover_data=hover_data)
fig.show()
Last, we show the first words of 10 articles from the cluster “0”.
# show articles in a specific cluster
cluster = "0"
df_subset = df[df["label"] == cluster].reset_index()
for i,row in df_subset.iterrows():
print(f"- {row['text_short']}")
if i == 10:
break
- NHLPA remains united in labour dispute CBC SPORTS ONLINE - Solidarity was the key word at Tuesday #3
- Lecavalier signs with Russian team, says Richards headed there <b>...</b> Vincent Lecava
- NHL, Players' Union Reject New Proposals (AP) AP - The NHL rejected last week's proposal by the play
- Stanley Cup Disappears in Airport Mishap (AP) AP - The Stanley Cup spent Sunday night in luggage lim
- #39;I see no hope whatsoever #39; CHICAGO -- There was no Chicago Hope yesterday. If there was any
- No faceoffs, just a standoff Barring a miracle (which isn't coming), the National Hockey League owne
- NHLPA won #39;t alter original proposal NHL Players Association executive director Bob Goodenow said
- NHL union head: Season in doubt NHL union head Bob Goodenow said Tuesday that locked-out players rem
- NHLPA #39;s proposal a small step in right direction Toronto, ON (Sports Network) - NHL commissioner
- Some fans cooling off, take NHL's hit in stride For hockey fans, this winter might just be the colde
- Hockey big men go head-to-head The lockout of NHL players by the league #39;s owners moved into its
Give Meaningful Names to Clusters#
Clusters named as “0”, “1”, or “2” don’t convey much about their content. Indeed, to understand what a cluster is about, we currently need to see some of its data as examples.
Let’s try to automatically give names to clusters that are related to the articles they contain. To do so, we can extract keywords from the texts from a specific cluster and then use them as the new name of the cluster. We use the KeyBERT
class to extract 10 keywords from the texts of cluster “0”.
# extracting keywords from texts with KeyBERT
cluster = "0"
df_subset = df[df["label"] == cluster].reset_index()
texts_concat = ". ".join(df_subset["text"].values)
keywords_and_scores = KeyBERT().extract_keywords(texts_concat,
keyphrase_ngram_range=(1, 1), top_n=10)
print(keywords_and_scores)
[('nhlpa', 0.5239), ('nhl', 0.5221), ('hockey', 0.4337), ('stanley', 0.3507), ('bettman', 0.289), ('cbc', 0.2888), ('hopes', 0.2486), ('lockout', 0.2447), ('tampa', 0.242), ('toronto', 0.2369)]
Some of these keywords may be sort of repeated, such as “olympics” and “olympic”, as the library doesn’t reason on the stem of the keywords. To avoid using repeated keywords, we write a small helper function that removes these duplicate cases and keep only the first n_keep
keywords left.
# keep only the keywords with different stem
def filter_keywords(keywords, n_keep=3):
new_keywords = []
for candidate_keyword in keywords:
is_ok = True
for compare_keyword in keywords:
if candidate_keyword == compare_keyword:
continue
if compare_keyword in candidate_keyword:
is_ok = False
break
if is_ok:
new_keywords.append(candidate_keyword)
if len(new_keywords) >= n_keep:
break
return new_keywords
keywords = [t[0] for t in keywords_and_scores]
keywords_filtered = filter_keywords(keywords)
print(keywords_filtered)
['nhl', 'hockey', 'stanley']
Following this example, now it’s easy to implement the logic that assigns a new name to each cluster.
# assign a meaningful name to each cluster
def get_cluster_name(df, cluster):
df_subset = df[df["label"] == cluster].reset_index()
texts_concat = ". ".join(df_subset["text"].values)
kw_model = KeyBERT()
keywords_and_scores = kw_model.extract_keywords(texts_concat, keyphrase_ngram_range=(1, 1),
top_n=10)
keywords = [t[0] for t in keywords_and_scores]
keywords_filtered = filter_keywords(keywords)
return " - ".join(keywords_filtered)
# get all the new cluster names
all_clusters = df_no_outliers["label"].unique()
d_cluster_name_mapping = {}
for cluster in all_clusters:
if cluster == "-1":
d_cluster_name_mapping[cluster] = "outliers"
else:
d_cluster_name_mapping[cluster] = get_cluster_name(df_no_outliers, cluster)
# rename clusters
df_no_outliers["label"] = df_no_outliers["label"].apply(lambda label: d_cluster_name_mapping[label])
Let’s plot the embeddings again, this time with the new cluster names.
# scatter plot
hover_data = {
"text_short": True,
"x": False,
"y": False
}
fig = px.scatter(df_no_outliers, x="x", y="y", template="plotly_dark",
title="Embeddings", color="label", hover_data=hover_data)
fig.show()
It’s more readable, right?
Clustering with BERTopic#
A similar clustering pipeline is implemented in the BERTopic library, which uses sentence embeddings, UMAP, HDBSCAN, and other algorithms.
Code Exercises#
Quiz#
What text better describes the UMAP algorithm?
A non-linear dimensionality reduction algorithm based on manifold learning
An unsupervised learning algorithm for clustering data
A supervised machine learning algorithm for classification
A deep learning algorithm for image recognition
An algorithm for identifying objects in videos
Answer
The correct answer is 1.
Which of the following is not the name of a clustering algorithm?
HDBSCAN
OPTICS
KMeans
Isolation Forest
Gaussian Mixture
Answer
The correct answer is 4.
What is a quick way of assigning meaningful names to clusters?
Using keywords extracted from their texts.
Tagging the clusters with random numbers.
Identifying the most frequent words in the clusters.
Answer
The correct answer is 1. The option 3 is wrong because stopwords are very likely to be the most frequent words in each cluster.
Questions and Feedbacks#
Have questions about this lesson? Would you like to exchange ideas? Or would you like to point out something that needs to be corrected? Join the NLPlanet Discord server and interact with the community! There’s a specific channel for this course called practical-nlp-nlplanet.